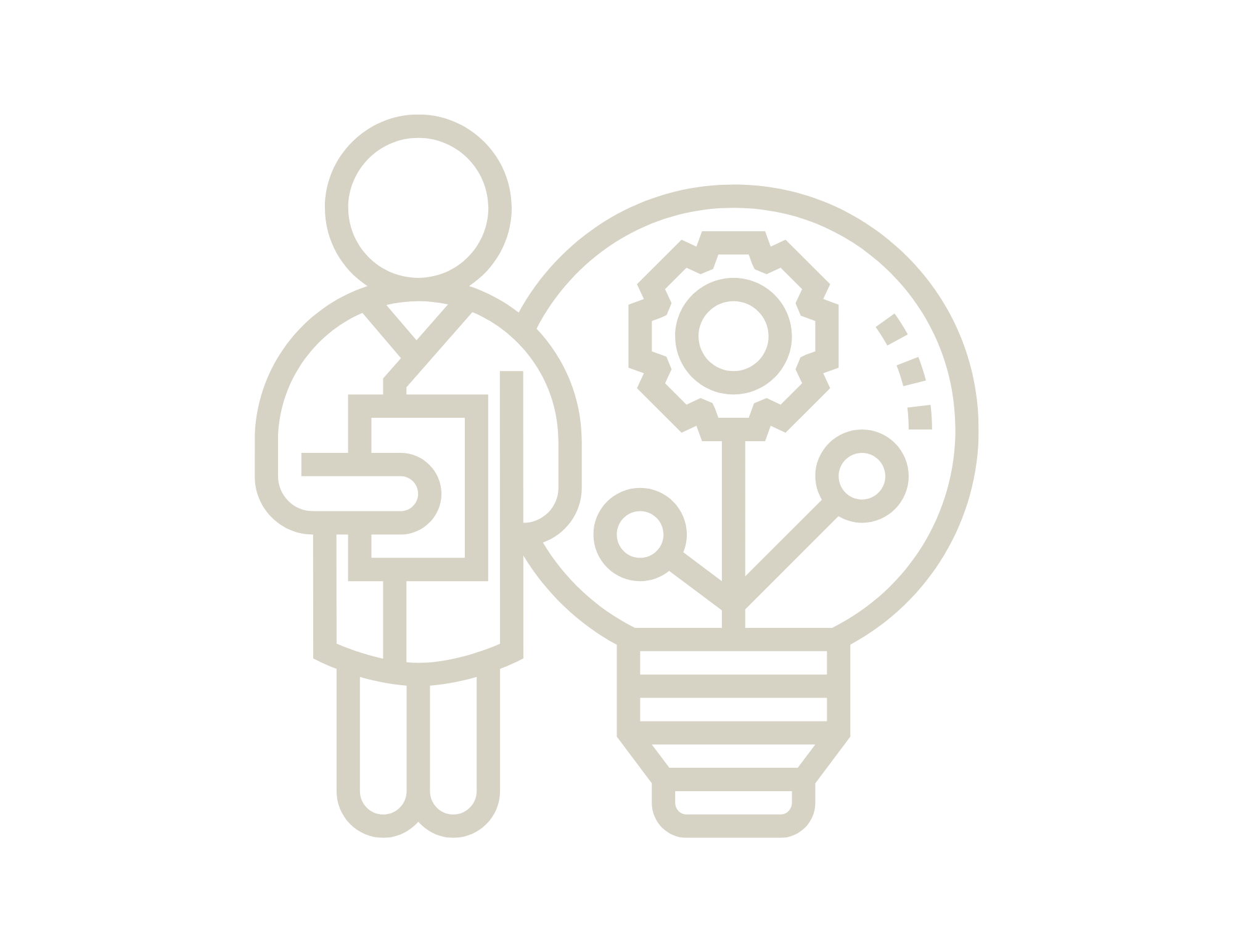
Our Research
Cheap and abundant energy has been essential to human development for the past two centuries. It has improved the quality of life and extended lifespans by enabling artificial environments, medical interventions, knowledge sharing, freedom of movement, and lighting the night.
The future of humanity depends on identifying and developing new energy sources. This includes global connectivity, advanced computing, and space travel. However, even though it is relatively easy to develop subsurface reservoirs, they remain challenging to model, predict, and optimize.
In partnership with ConocoPhillips, the goal of the Energy Analytics stream is to use advanced analytics, machine learning, and spatial statistics to help predict, develop, and optimize energy sources -- to ensure a sustainable future for all.
Energy Analytics Projects
- autonomous well trajectory planning
- 3D Kriging Python module
- neural ODE generative network
- formation top depth recommender system
- subsurface data munging
- novel visualizations of well completion data
- well completion imputation
- generating geologic maps from satellite images
- automated well log mnemonic aliasing
- generative networks for denoising images or human free subsurface interpretation.
- high resolution StackGAN architecture
- recommending oil well acquisitions
- removing structured noise from satellite images
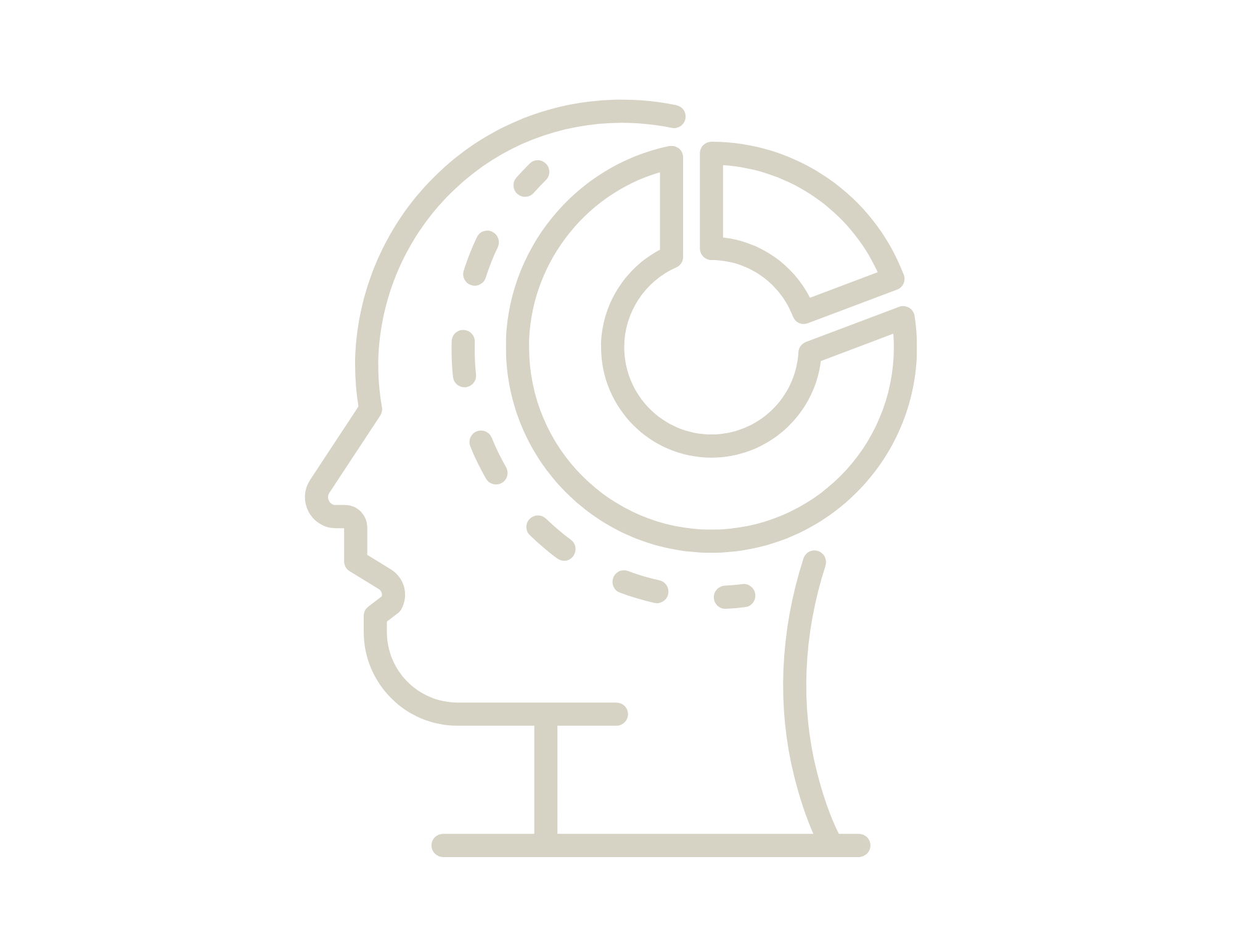
Our Strategy
In Energy Analytics, we partner with ConocoPhillips to help solve subsurface energy challenges using data anlytics, spacial statistics, and machine learning. Our projects are all implemented in Python and span topics from well design to interpolation and maximizing production. Our stream is focused on applied research that will help our partners in the energy industry.
We are grateful for the support from our sponsorship from:
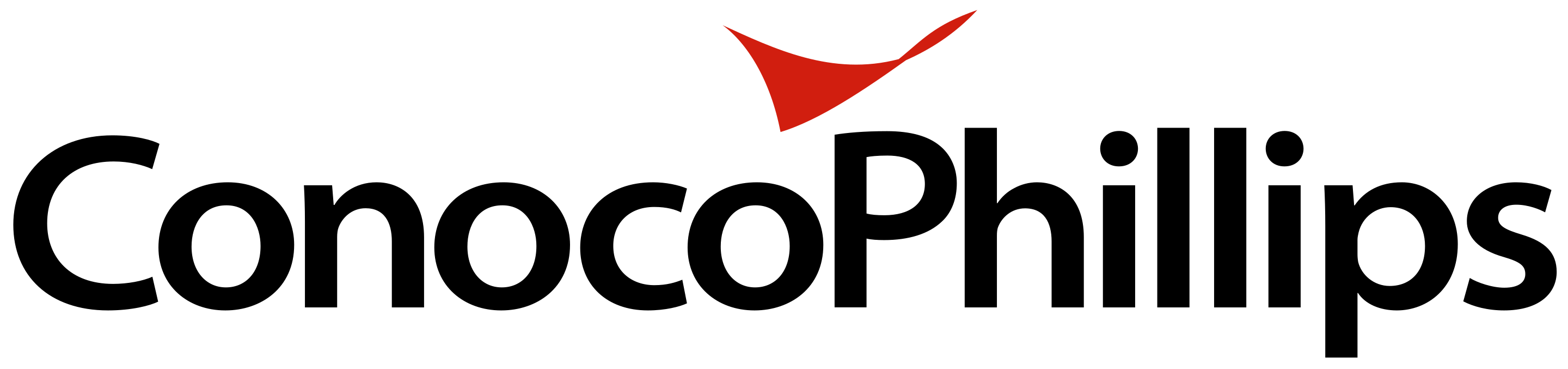
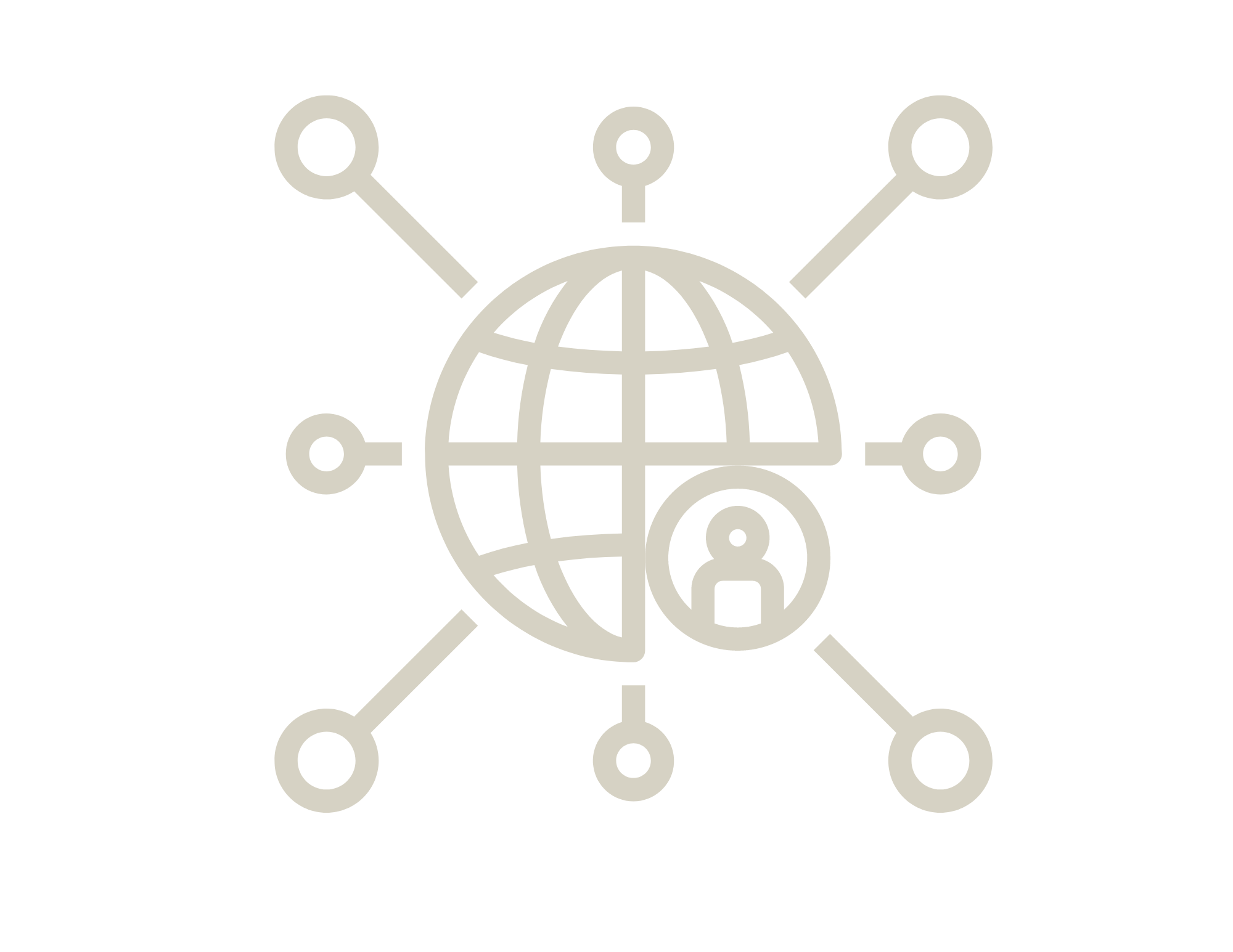
Our Impact
Recent numerical developments and improved computational resources have led to a rapid expansion of big data analytics and machine learning implementations. These technologies are disrupting many industries. Oil and gas has a long history with big data from seismic surveys, production monitoring, remote sensing and well-based data. Additionally, there are various physics-based engineering and stochastic statistical workflows. There is an opportunity to adapt and tool big data analytics and machine learning to optimize subsurface development to maximize the value of national energy resources and to minimize environmental impacts. We are directly contributing to applied research in energy analytics at all stages with advanced skill sets and techniques.
Resources
Course Credit
Research Outcomes
ConocoPhillips also partners with the Energy Inventors Program Practicum, providing a set of curated problem statements for students to work on over the course of a single semester.